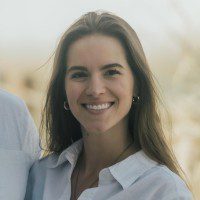
Samantha Rohn
On November 30, 2022, OpenAI released ChatGPT, its generative AI large language model powered by GPT-3, into public availability.
Within weeks, it racked up 100 million users, spawned an entire ecosystem of applications, plugins, and products designed to help users access GPT in a refined format, and started raising concerns about everything from AI-generated deepfakes to the existential risk artificial intelligence poses to humanity.
Among other astonishing capabilities, language models like ChatGPT can:
ChatGPT has introduced generative AI to knowledge workers and has started conversations about using generative AI models to automate manual work. This provides endless use cases for customer support challenges, where interactions and requests tend to be repetitive, but with nuance that can be easy to miss.
This article will help you understand the impact generative AI is already having on customer support, explore use cases where generative AI can transform your customer support, limitations to watch out for, and an actionable step-by-step guide to make it happen for your customer service and support team.
Generative AI refers to artificial intelligence that creates human-like content from scratch—images, videos, music, and text. The most common applications of generative AI are large language models (LLMs), which use deep learning algorithms to analyze vast amounts of text to learn how human language is structured and generate unique content ‘inspired’ by its training corpus.
Generative AI is most helpful as a second brain that can digest large amounts of data and render it back upon request—which makes it a valuable and disruptive tool for customer support.
Language models can be trained on (or granted live access to) your product’s database, customer conversations, brand guidelines, customer support scripts, and canned responses to ‘understand’ customers’ needs and resolve their queries.
Nowadays, brands use chatbots to segment tickets by issue. But when customers can’t identify which bracket theirs falls into, they just add it to the general firehose. Categorizing tickets manually can be tedious, especially when coupled with the responsibility of resolving customer issues.
Large language models can be trained on all your support tickets to date to ‘learn’ where to classify specific queries based on the words referenced against previous tickets. It can also create an algorithm to automatically segment your support tickets into priority levels.
Unlike traditional chatbots that need every detail specified with “if/then” logic, generative AI chatbots and digital assistants can handle basic queries by interpreting them and referencing the data requested against the database it’s trained on. This beats the typical chatbot workflow that requires customers to go through an elimination process to narrow down their question.
Better still, if you grant a generative AI model access to your live database, it can go above and beyond by answering dynamic questions that have variable answers, such as:
These digital assistants enable end-users and provide customer self-support that provides a better overall customer experience, reduces time-to-resolution, and deflects support tickets.
Shared inboxes help support teams collaborate on customer conversations and reassign conversations internally based on expertise or variables such as who previously attended to a specific customer. A generative AI model can take that sorting and routing process a notch higher by automatically assigning tickets to team members based on their:
This improves the efficiency of support-related processes and activities, accelerates resolution, and enables SMB to enterprise support teams to manage support ticket queues more effectively.
With customer feedback, either you’re getting too little or too much—there’s no middle ground. While a startup might get 1:1 feedback from 50 customers, it’s often too little to build a truly transformational product. And while a billion-dollar enterprise can quickly rack up millions of reviews, ratings, and suggestions across its channels (Play Store, G2, in-app surveys, etc.), who do you think will read through all of them?
Your dedicated generative AI support model, that’s ‘who.’ More than just capturing the title and ratings from reviews and comments left by customers, LLMs can help you digest them at scale and identify issues with your product that are raised repeatedly by customers.
While generative AI might not be able to completely replace human agents just yet, it augments your support team’s capabilities and helps them automate repetitive, mind-numbing work, process and validate large volumes of data, and answer repetitive queries and tickets. Here are some of the benefits you can expect when you start integrating generative AI into your support operations.
How long, on average, does it take your support agents to hold a conversation and resolve the customer’s issue from start to finish? That metric is known as your average handle time and is used to measure agent productivity. According to Talkdesk’s 2021 Global Contact Center KPI Benchmarking Report, the figure ranges from 3:11 for hospitality brands to 4:13 for manufacturers.
Ultimately, average handle time is something of a paradox—the more calls your agents can cram into a day, the better. However, if they push it to the limit, customer experience might suffer. So, balancing speed and quality conversations is basically impossible without hiring more agents.
That’s where generative AI models can help. Given enough computing power, generative AI assistants can suggest answers, generate call center scripts, draft emails, and sort and categorize conversations infinitely without a break. Of course, you might want a human in the loop to vet and edit AI-generated answers lightly, but being able to consult a second brain that works at the speed of thought 24/7 is a game-changer.
If you grant it access to your customer database, an LLM can use customer data, such as purchase history and demographics, to customize help experiences, offers, and follow-ups better than a human agent can. With a sufficiently large trough of data, generative AI-powered support engines can suggest complementary purchases, seasonal gifts, discounts, etc., customized to individual customers.
As your business scales internationally, an increasing number of your customer tickets will come in outside normal working hours. Most businesses try to surmount this by hiring a distributed team of customer support managers so that there’s always a live support agent(s) to respond to tickets, but the costs can be prohibitive as you scale.
Generative AI solutions can be used to generate email replies, chat conversations, and step-by-step walkthroughs that explain how to resolve known issues. Even if you decide to keep a human in the loop to vet AI-generated answers, it’ll cost you significantly less than you’d have spent trying to build a globally distributed team to offer 24/7, real-time support.
HUBSPOT
Generative AI can be used to offer customers personalized advice and follow-ups regarding the type of product/configuration they’ve purchased so they can make the most of it, avoid common mistakes, and understand how the product works without contacting support. This way, you can educate customers and provide proactive customer support to preempt known issues before they raise them.
The Society for Human Resource Management estimates that training a new hire can cost up to $4,129. For support roles, that figure jumps to $10k – $20k per head when you factor in 1:1 coaching, courses, and on-demand resources required to get your staff up to speed.
With all that investment, support teams have some of the highest attrition rates that can peak at 87.6%, according to this Cresta Insights report. Outsourcing isn’t a better idea either, since you’ll be spending $2,600 to $3,400 per agent per month on contractors.
By comparison, an analysis by SemiAnalysis shows that OpenAI’s ChatGPT costs just $0.36 per answer—and it’ll only get cheaper as newer models that use computing power more efficiently are released.
Unlike the outlay required to hire, train, and manage human agents, generative AI models can be deployed in hours and with negligible computing costs, whether you’re a five-person startup or a Fortune 500 company. Even if you decide to host a private instance for privacy, it’ll still cost an order of magnitudes less to train an LLM on your data and integrate it with your CX platform than it’d cost to grow a support team.
Customer satisfaction and retention are more of a derivative that you achieve by doing lots of things right, and there’s no one lever you can pull to double repeat purchases and positive word of mouth overnight.
Every time your customers get their issues resolved quickly, without having to wait on hold, go back and forth with a human agent, repeat their request multiple times, or be transferred between agents (thanks to your generative AI chatbot and assistants), it leaves a positive impression that builds customer loyalty.
GPT-4 (the model most generative AI assistants are built on) can answer multi-choice answers in 26 languages, ranging from Welsh to Ukrainian, while DeepL, a competing AI-powered translator, can translate text between 31 languages, including Slovak, Turkish, and Latvian. Generative AI translators can help support teams communicate with international customers and localize help resources in their audience’s preferred languages without growing headcount significantly.
Quoting Alphabet (Google’s parent company) CEO Sundar Pichai, “Artificial intelligence will have a more profound impact on humanity than fire, electricity and the internet.” But, humanity’s history with the trio of fire, electricity, and the internet has been bittersweet, with stratospheric highs and low lows.
Generative AI raises privacy concerns, lacks the personal touch, and non-sophisticated models can struggle with handling complex, non-linear queries that require a human in the loop to triage and understand a customer’s intent.
As of July 2023, ChatGPT hasn’t even been out eight months in the wild, and it’s already getting banned left and right—companies like Apple, Samsung, Verizon, Accenture, and a slew of banks such as J.P. Morgan Chase, Bank of America, and Goldman Sachs have banned internal ChatGPT usage due to the risk of data leaks.
In Samsung’s case, an employee pasted code from a faulty semiconductor database into ChatGPT to ask it for a fix; likewise, another worker shared confidential code with the LLM to help them find a fix for a defective device.
LLMs like OpenAI’s GPT (which ChatGPT is built on) feed on data and add conversations with users to its corpus to generate even better replies. If your employees are feeding confidential IP into ChatGPT, that’s obviously a problem that creates an opportunity for loss of IP and future litigation.
Despite the large corpus of facts and answers it can generate from its training data, LLMs like GPT-4 can’t empathize with customers.
Don’t get this wrong—ChatGPT can apologize, ask you to be patient, and even make a few jokes to lighten your mood. But, ChatGPT doesn’t understand the frustration of buying a laptop from Amazon to find its screen was broken in transit; it can’t really relate to clearing your cache, deleting cookies, restarting your computer, reloading a website, etc., without still being able to sign into an application.
It’s incapable of relating to that lived experience, and while it can do its best to engage customers, a real-life customer agent will always take the cake on the emotional intelligence angle instead of just trying to provide a fix and end a conversation ASAP.
Growing up, we all got a stern talking-to for making up things. So, what are the penalties if you simply make up cases that don’t exist for a legal battle? New York lawyers Peter LoDuca and Steven Schwartz found themselves in that situation when they submitted a 10-page brief featuring six prior court decisions that had all been made up by ChatGPT.
They all looked completely convincing, with names, dates, precedents, etc. But, they were all made up. OpenAI’s GPT model doesn’t regurgitate information word-for-word; it aims to find patterns in the data it’s trained on, ‘digests’ it, and reconstructs them when prompted.
This creates situations where it hallucinates nonexistent facts that are based structured to look convincing, just like in the aforementioned case. LoDuca and Schwartz got off with a $5,000 fine, but on a large enough scale, generative AI models can make blatantly misleading claims about your brands, products, and services, especially if there’s no human in the loop. You always need to vet answers, except for basic queries that require linear, straightforward replies.
Just like in the aforementioned legal case, generative AI models can make your support team hopelessly dependent on technology—initially, your experimenting with AI starts innocently enough with tight oversight. But, as your employees get more comfortable with its functionality, it’s easier to share confidential data and not vet AI-generated output.
That’s when you might start seeing an uptick in hallucinated or even false answers driven by poor internal controls.
Most customers want to interact with customer service as little as possible. Even when it’s necessary, they treat it like a colonoscopy—the shorter it takes, the better. So, this particular segment won’t make exceptions to being attended to AI-powered experiences as long as they work well and have a human in the loop to right the ship if anything goes wrong.
Some other customers might have reservations, either due to ideological reasons (“AI is taking jobs away!”), wanting to speak to an actual human, or even wanting to play around to get it confused. The key is to fully disclose when a customer interaction is AI-generated and offer alternatives customers can use if they feel they’re not getting the help they need quickly enough.
So, use generative AI, but don’t make it an obstacle to the help your customers need.
Generative AI models often struggle with short-term memory—they can reference gigabytes of data stored long-term, but are often unable to carry on a single line of thought without getting ‘confused.’ This can be a significant issue when a customer submits a long-winded ticket that needs to be broken into bits to be understood and might require a collection of fixes.
For instance, a generative AI assistant will likely not struggle with a ticket asking:
It’ll simply reference a support article or a delivery tracking database and offer a straightforward answer. But, if a customer tries to describe a technical issue and the steps they’ve taken to remedy it, your generative AI assistant will possibly get confused or suggest a wrong, next-best answer, especially if it doesn’t have a human in the loop to take over or edit its replies.
SUNDAR PICHAI
CEO, ALPHABET
Before you launch your generative AI pilot project, you need to specify your goals, the parameters you’ll track to measure success, and a timeframe for your experimentation. Your goals might be to reply to support requests faster, reduce wait times by at least X%, increase customer satisfaction, and enable more customers to resolve issues independently with self-help content.
To track the success of your pilot program, you need to specify customer experience metrics and KPIs to track, such as NPS, CSAT, customer effort score, time-to-resolution (TTR), average handle time, and churn.
While you specify the metrics and KPIs your support team will track, you need to equally set performance benchmarks by studying historical data from previous customer support interactions.
For instance, you need to know how long customer conversations take on average (using average handle time and TTR), gauge your customers’ sentiment (using the Net Promoter Score methodology), or even conduct 1:1 interviews to understand where your customers think your support experience can be improved.
Here’s where you have to choose between buying or building your generative AI experience from scratch. Major CX and help desk platform players like Zendesk, Intercom, and HubSpot have already begun integrating AI assistants into their products so that you can train and deploy them on top of your help articles and knowledge bases. If you prefer, you can directly integrate with the API of OpenAI or similar services like Claude or Google Bard. This will allow you to customize and build a solution that is tailored to your specific needs and can be more closely integrated with your internal tools.
If you’re going with a pre-integrated generative AI assistant (from Zendesk, Intercom, HubSpot, etc.), you may be able to skip this step since your customer conversations and help library live on the same platform, which your AI assistant has easy access to. If you’re going the in-house, DIY route, here’s where you need to collect and tag conversations, canned responses, and help articles for training your AI assistant; you’ll also need to remove any personally identifiable information or sensitive data before feeding your data into the model for training.
Training a generative AI model can be broken into several steps, including:
After training, you’ll need to validate your generative AI assistant in a controlled environment, possibly by opening it up to your internal support agents or a smaller segment of customers. Your goal here is to track the performance metrics (AHT, CSAT, NPS, TTR, churn, etc.), collect live user feedback, and gradually eliminate performance issues. If you’re on a tight timeline, you can block your model from entertaining certain requests completely, editing or refining tone, etc., to make your generative AI assistant more engaging and professional for rollout.
Again, if you’re using a packaged generative AI support solution, this isn’t a problem because of the tight integration with your CX data (on which your assistant is trained) and your conversational channels (live chat, email, shared inboxes, etc.) platforms like Zendesk and Drift offer.
But, if you’re building a custom solution, here’s the stage where you integrate your AI model side-by-side with your support team’s tools, including messaging, help library, etc.
As your generative AI model goes into general availability, you’ll uncover more bugs, errors, and exceptions in the wild. But, you can think of the post-deployment stage as more of an iterative learning process where you observe, refine, and update your generative AI capabilities to fit your agents’ workflows and answer customer queries more accurately.
Whatfix offers a guided adoption solution for support teams and organizations making generative AI a part of their support workflow. The platform acts as a handy addition to your AI-enabled support system and helps your customers understand how to interact with your product, refine queries for your AI assistant, and avoid known errors.
Whatfix augments your generative AI rollout using:
Learn more by setting up a Whatfix demo today.
Thank you for subscribing!